Today, data governance projects are becoming more and more important as they are increasingly focused on production, storage, distribution and exploitation methods.
This race for data and the three V’s of Big Data (Volume, Variety, Velocity) leads organizations into a data governance that is sometimes irrational and rarely profitable. Finally, data as it is managed today poses as many problems as it provides solutions to its users and is now well and truly obsolete. It is therefore necessary to enter the information age, announced decades ago and now within everyone’s reach.
Interconnecting heterogeneous systems
In an ecosystem where needs are evolving more and more rapidly, heavy monolithic and inflexible applications are being replaced by more flexible applications that are open to their environment through various APIs. Beyond their primary missions centered on usage, these applications gain value through the production, storage and exchange of data in the restricted perimeter that is assigned to them (production of logs, capitalization of a pipe antenna, capitalization of health data, collection of legal contracts, various AI modules…). This diversity of applications brings real added value to processes and their interconnections therefore become a major challenge for companies wishing to derive maximum benefit from the collaboration of these applications, because it is from the connection between the data that truly relevant and exploitable information for the end user is born.
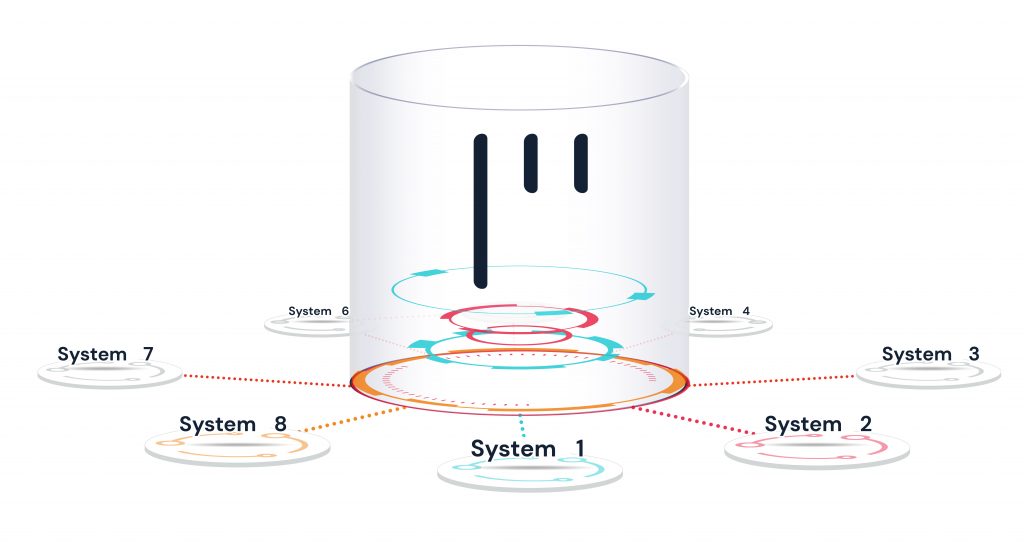
But to be truly effective, it is not enough to interconnect data, it is necessary to be able to extract meaning and contextualization. At present, only semantic technologies can achieve this level of interconnection.
Obsolete Data
In order to illustrate the issue of data/information exchange, put around the same table two people who both speak different languages: an Englishman and a Frenchman. If neither of them is bilingual, they can’t communicate, and therefore they can’t exchange information and understand each other. They then call on translators to translate point to point from English to French, and from French to English.
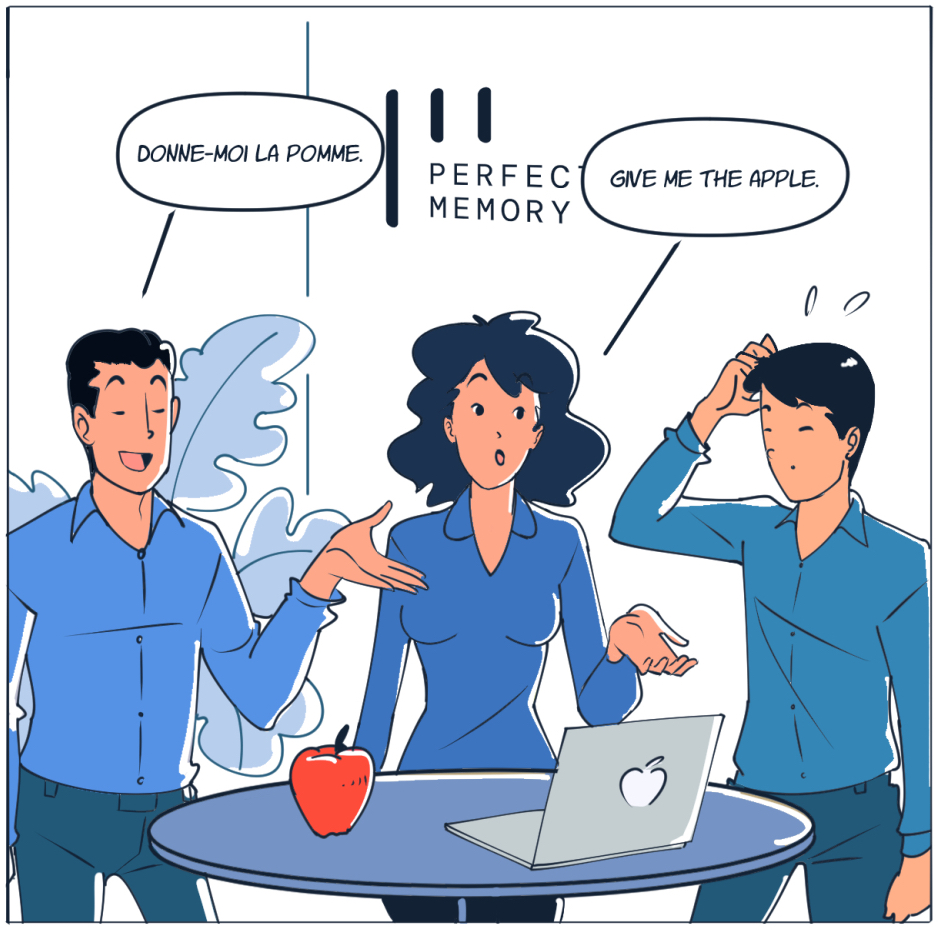
Now imagine adding new interlocutors all speaking different languages. The combination of translators to insert quickly becomes a problem.
Even today, the vast majority of data collection and management systems operate on the same principle. The interconnection of two heterogeneous systems implies either the implementation of a data structure mapping allowing the exchange of data from one system to another (like an xslt, ERP transformation, …) or the use of a standard data schema which is authoritative and with which the two systems are compatible (everyone speaks English to the detriment of cultural specificities, structure and therefore thinking), .
Beyond the scalability issues that arise as soon as the number of systems to be interconnected grows, the transformation of a symbol into another symbol poses an even greater limit: that of interpretation.
Let’s say the French person says « pomme« . The FR → EN translator will then translate « apple« . The English speaker may then interpret the symbol « apple » as the company Apple, whereas the French speaker is talking about fruit here. This trivial example shows a very important thing: the transformation of a symbol into another symbol is not enough to guarantee the exchange of information. The real job of a translator is not to transform a symbol into another symbol, but to extract the meaning of the symbol before rendering it into the correct language.

The same is true for a data mapping operation between two heterogeneous systems. A mapping from one symbolic structure to another symbolic structure will never be sufficient because it will never be scalable and always limiting in terms of intelligibility for the machine, and a fortiori for the users. The same is true for traditional AI modules, which are more and more a source of disappointment for users who find themselves accumulating large amounts of data out of context and of dubious usability.
To overcome this state of affairs, it is necessary to enable real collaboration between systems, by allowing them to collaborate in a common language, and therefore, rather than sharing common symbols, to share common concepts within a repository, in the same way that Apple is an edible fruit from a tree and that Apple is an American organization with its headquarters in California.
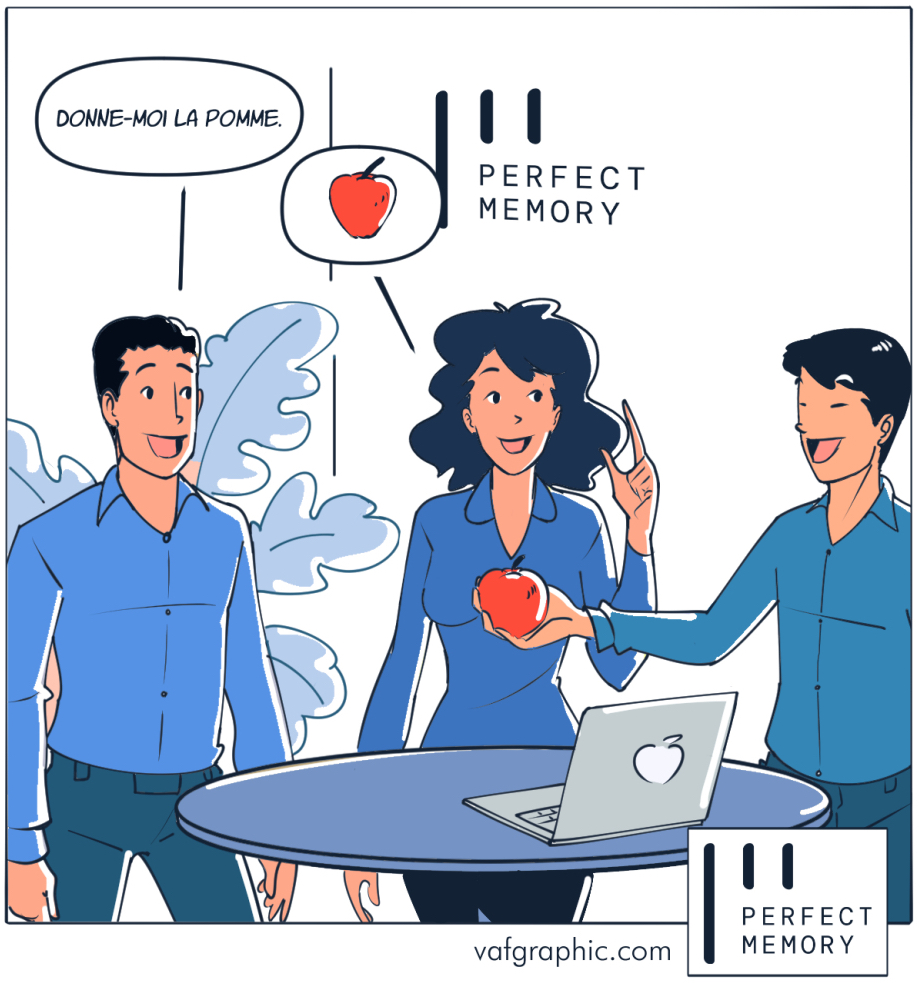
The advent of information
For two people who do not speak the same language to understand each other, it is therefore not enough to translate symbols, but to share common concepts. Semantic technologies give machines the ability to share common concepts through a repository called an ontology : a model that relegates data and symbols to the level of simple representations to be displayed on the screen, in favor of structuring intrinsic information. The machines that use this semantic model now produce, store and share information, i.e. the fruit of the interpretation of a set of data. It is no longer raw data that circulates between agents, but information that is directly usable and intelligible for business teams, thus considerably reinforcing their understanding and control of the organization’s processes outside of any technical considerations.
In a conventional (non-semantic) computer system, this interpretation is performed by the human brain. The machine displays the term « David Bowie », the human end-user deduces, based on the context and a common culture, that it is the British singer and author of Space Oddity.
In a semantic system, it is the machine that is directly capable of collecting, storing and processing information, and not just data. It is therefore able to abstract a set of data via semantic elevation mechanisms in order to extract information without imposing a particular structure on the source applications.
Information for knowledge
The interconnection of heterogeneous systems in the same environment through a semantic platform thus allows the collection and linking of thousands of pieces of information which, once associated and contextualized, can themselves produce new information, through semantic reasoning.
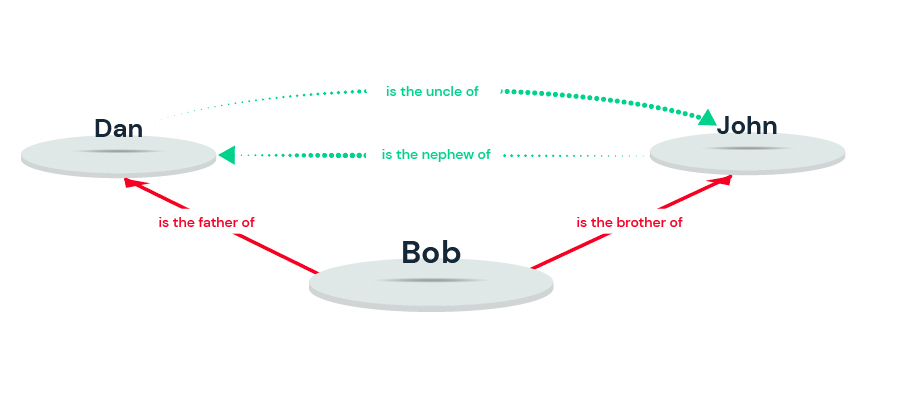
This reasoning mechanism, which is very intuitive for a human brain, is much less so for a machine. However, it is becoming a reality for semantic systems capable of integrating the rules dictated by the businesses specific to the context in which they operate.
It is important to note that in semantic systems, this reasoning capability is implemented directly at the semantic database level, and not in ancillary scripts that require additional execution. This means that everything that constitutes the knowledge of the organization, the information, the business rules and the deductions that result from them, are all centralized in the same place, in the central memory of the organization.
Knowledge as the basis for a Perfect Memory
Finally, the use of a semantic platform allows the collaboration of a set of heterogeneous systems, whether endogenous or exogenous to the organization, and makes it possible to build an organizational memory. This memory contextualizes and centralizes all the knowledge produced by an ecosystem in one place. It fulfils all the conditions of a perfect memory:
- it is intelligible to users and other applications connected to the platform
- it is relevant to its context of application
- it is operational
- it becomes accessible to all
- it is alive, because the stored knowledge as well as its common repository (the ontology) can evolve at any time according to the changes of the operational realities
- it also deals with the mechanics of forgetting, a necessary condition for an exercise in intelligible thought, as Jorge Luis Borges formulated it in his short story « Funès or Memory
In this respect, we at Perfect Memory are convinced that enabling machines and processes to collaborate at the level of information rather than raw data is becoming the necessary condition for streamlining data governance policies and manipulating what is really relevant to business teams: intrinsic knowledge.